Background
A group of scientists within the Fraser lab have begun a journal club centered around issues of diversity, equity, inclusion, and justice within academia, specifically in the biological sciences.
Our goal is to provide an environment for continued learning, critical discussion, and brainstorming action items that individuals and labs can implement. Our discussions and proposed interventions reflect our own opinions based on our personal identities and lived experiences, and may differ from the identities and experiences of others. We will recap our discussions and proposed action items through a series of blog posts, and encourage readers to directly engage with DEIJ practitioners and their scholarship to improve your environment.
June 10th, 2022 – The STEM Pipeline
Discussion Leader: Chris Macdonald
Articles:
- Problematizing the STEM Pipeline Metaphor: Is the STEM Pipeline Metaphor Serving Our Students and the STEM Workforce? Cannady MA, Greenwald E, and Harris KN. DOI: 10.1002/sce.21108
- Reimagining the Pipeline: Advancing STEM Diversity, Persistence, and Success. Allen-Ramdial SAA, and Campbell AG. DOI: 10.1093/biosci/biu076
- Improving Underrepresented Minority Student Persistence in STEM. Estrada et al. DOI: 10.1187/cbe.16-01-0038
Bonus Article:
Planting Equity: Using What We Know to Cultivate Growth as a Plant Biology Community. Montgomery BL. DOI: 10.1105/tpc.20.00589
Summary
STEM graduates require extensive education, and progressively demand more specialized and advanced training. This has some implications for DEI work. One important one is that each educational level has compounding effects on the following ones. The common metaphor of a “STEM pipeline” has been used to capture this idea, where learners who move away from a STEM career trajectory are the leaks. In a DEI context, this means differential leakiness would be important to consider.
Metaphors can be useful by simplifying complex systems and helping us reason about them. That assumes they accurately capture the important dynamics of the system, however. If they don’t they can hinder our thinking. Some have claimed that the pipeline metaphor is such a case, challenging both its accuracy and the helpfulness of the interventions it suggests.
I picked these three papers because they critically evaluate the value and accuracy of the metaphor and suggest policies to achieve the outcomes we want (a diverse and equitable environment) but that might not come directly from thinking about leaks.
-[Cannady et al.] uses longitudinal data on students in the US to see if the metaphor is accurate, and claims it is not.
-[Allen-Ramdial et al.] builds off the inaccuracy of the metaphor and suggests policies that the “pipeline” might not suggest
-[Estrada et al.] is a product of the Joint Working Group on Improving Underrepresented Minorities (URMs) Persistence in Science, Technology, Engineering, and Mathematics (STEM), which was convened by NIGMS and HHMI. It is an example of how a large working group can adapt the criticisms of the previous two papers and propose policies to achieve an equitable environment.
As I was picking the papers for our discussion, I also thought about alternative metaphors we might use and whether they would help us think differently. I discovered the article by [Beronda L. Montgomery], which offered a wonderful example of a very different way of thinking about education that would lead us to do different things as a result.
Key Points:
- The metaphor may not be accurate: similar numbers of underrepresented minority students and non-underrepresented minority students enter STEM majors, and similar proportions remain through undergraduate education.
- The metaphor leads us to think that trajectories are strictly one way (you can’t unleak), while in fact there is much more fluidity in practice.
- The metaphor focuses our attention on individual failures (the leaks) rather than institutional ones (the pipes).
- There is an important distinction between an institution’s culture, which is essentially the beliefs, policies, and values that guide behavior, and its climate, which is the result of the actual implementation of them. An institution may have an unwelcoming or harmful climate while still having a healthy culture, but the pipeline metaphor focuses our attention on policy rather than implementation.
Open Questions:
- Is “STEM” a useful category, or is it too broad?
- What sorts of trajectories do “typical” successful scientists follow? What is the definition of “success” in STEM?
- What differentiates “leaky” institutions from others?
- How can we take the useful features of the pipeline metaphor and avoid the harmful ones?
- How does the overall educational landscape influence DEI efforts at the post-secondary levels and beyond?
Proposed Action Items:
We broadly agree with the policies suggested by [Allen-Ramdial et al.] and [Estrada et al.], although they are larger-scale interventions. In particular:
- Engage across institutions. Faculty at minority-serving institutions play essential but often ignored roles in diversifying STEM, and DEI initiatives at research-intensive institutions sometimes only engage with other research-intensive institutions. Programs that connect faculty across institutional boundaries can contribute to diversifying trainee access to career opportunities.
- Focus on aligning culture and climate. Ask how students and trainees feel, and listen to them. A failure of good intentions may be a result of both culture and climate.
- Take faculty involvement in DEI seriously. Effective and long-term DEI efforts are much more useful than broad but shallow activities. Institutions can encourage deep engagement by evaluating faculty DEI work on par with teaching and research.
- At an individual level, we found rethinking our metaphors can be a useful exercise. Ask yourself: what sort of environments would I like to create? Are the concepts I deploy sufficient to get there? Are they accurate? Are there alternatives?
Over the past two years I have done a bunch of structural bioinformatic work, resulting in the paper Ligand binding remodels protein side chain conformational heterogeneity. And I made A LOT of mistakes.
Below are many of the lessons, guidelines, and pitfalls for a structural bioinformatic analysis. While many of the principles below are specifically tailored to a paired analysis (such as apo versus holo or peptide bound versus small molecule bound), these guidelines can help with any structural bioinformatics project.
For specific suggestions, I have the code I created linked at the bottom of each section. This code is built on bash, python, Phenix/cctbx, and qFit. The code should be easily adaptable to other projects/inquiries. If there are any questions, feel free to contact me.
Define your selection criteria early.
Before you start downloading structures, you need to decide what structures you would like to highlight. Some of these items can be subsetted using the PDB advanced selection criteria, including:
- Method of structure (X-ray, CryoEM, NMR, Neutron, ect)
- Resolution
- Cryo or Room Temperature
- Size of the protein
- Type of ligands
- Single or multidomain proteins
You may also want to cross check these structures with external databases (ChemBL, Uniprot, ect). You can do much of this work on the PDB website in their advanced search section.
Once you get a list of structures with your initial criteria, you can parse the header of the PDB or get other statistics of PDB/density file from the MTZ file with a program like phenix.mtz_dump.
This is the stage where you will start creating pairs of structures. Some criteria you will want to think of at this stage include:
Unit cell dimensions and angles
Space group
Sequence (get this from the PDB and not from another database to know which residues were actually resolved in the structure).
Ligand types/crystallographic additives (how much overlap do you want between the paired structures)
Experimental methods such as crystallographic conditions (this will be tricker but may be important and worth it to go through headers manually).
At this stage, I suggest keeping duplicate pairs (ie if you have multiple apo or wildtype proteins for each holo or mutant proteins). Many structures will be thrown out downstream and it can be helpful to have ‘back ups’.
Here is a pipeline you can use to select the PDBs to move forward in your analysis.
Re-refine structures.
The PDB has a lot of structures refined with many different software packages and versions. To ensure that you are comparing apples to apples, pick one refinement software version and re-refine all of your structures.
The software that I used was phenix.refine.
Unless you know exactly how you want to refine your structures, spend some time with ~15 structures and play around with refinement strategies. Some things to think about:
Do you want different resolution cutoffs to have different refinement strategies?
Are you going to refine anisotropically or with hydrogens?
How are different refinement strategies impacting the R-free or R-gap of structures?
Once you have a refinement script you are happy to test your refinement script with ~50 PDBs, find errors and adjust from there. As the PDB files you are feeding your refinement strategy may be labeled in many different ways, you are likely going to have to build in flags as well as if statements to refine the structures.
If 80% of your structures are re-refined, move on. Send bugs to the respective software groups, and accept your losses (trust me, they are not worth it!).
Here is an example re-refinement pipeline that works with Phenix version 1.19.
Here is a pipeline that will re-refine your structures, run qFit, and then refine your qFit structure.
Quality control of structures.
The first, and easiest part of quality control of structures has to do with refinement metrics.
Are you decreasing the R-free or R-gap? This can be extracted through refinement log files or running additional analysis.
Are there any clashes in the structure?
Are there Ramachandran outliers?
With pairs, you want to assess how well they align together in 3D. Aligning them is a beast in and of itself. Due to structures with the same sequence having different chain ids or residue numbers, we will need to match those up as all downstream analyses will rely on this.
There are many different methods to align structures, but I landed with pymol, alpha carbon align. This did not work well for 100% of the paired structures I had but it is what worked for the majority of them.
I then required all chains start with residue 1. Then, as I was working with paired structures, I based all holo structures off of apo structures. Therefore, I reassigned the closest geometric chain in the holo to the chain in the apo.
Some additional criteria you will want to think about in this stage include:
How well do the backbones of structures line up? This can be assessed by alpha carbon RMSD between the structures. While some analyses may want to keep large changes, others may want to throw them out.
How much do the ligands overlap between the structures?
Here is a pipeline that will extract and compare R-values, align your pairs, and spit out alpha RMSD and ligand overlap between the pairs.
Analysis of structures
Now we get to the fun stuff!
Before we can run any analysis, you need to think about how you want to extract information from the structures. Are you going to do it based on chain and residue numbering or based on location. I choose the former as it is easier downstream. However, this required me to reassign the chain and residue numbers for many structures (see above).
The other thing to think about when comparing structures is if there are duplicates. In my case, I had multiple holo assigned to multiple apo. Therefore in the analysis, it was important to keep track of not just the PDB, but also the PDB’s matched pair.
Finally, you also need to consider how you are going to look at certain sections of the PDB. For example, I wanted to examine binding site residues. But my criteria (any residue heavy atom within 5A of any ligand heavy atom) sometimes gave me one or two different residues in the holo or apo depending on how much those residues moved. I decided to look at the union of those two lists, but you could also look at the intersection of those two lists.
Here are a bunch of analyses that I ran on my pairs or individual models.
Quality control of the analysis
For almost every single analysis I did, I would plot the result and have a few outrageous outliers. This was always a clue of something I coded wrong in the analysis, or something incorrect about the labeling of the PDBs.
When looking at the result of your analysis, always look at the minimum and maximum values on both an individual basis (ie if you are looking at some sort of residue metric), as well as on a structure basis. Take at least the top and bottom five metric values and go through checking for the following:
Is residue 1, chain A of structure 1 within your RMSD cutoff of residue 1, chain A of structure 2?
If you manually calculate the metric you are measuring, is it matching what your code says?
Look at the structure in Pymol or Chimera. Does the numerical value of the metric line up with what you are visualizing?
Repeat this process until you can visually/biologically explain at least the top and bottom five metric values.
Background
A group of scientists within the Fraser lab have begun a journal club centered around issues of diversity, equity, inclusion, and justice within academia, specifically in the biological sciences.
Our goal is to provide an environment for continued learning, critical discussion, and brainstorming action items that individuals and labs can implement. Our discussions and proposed interventions reflect our own opinions based on our personal identities and lived experiences, and may differ from the identities and experiences of others. We will recap our discussions and proposed action items through a series of blog posts, and encourage readers to directly engage with DEIJ practitioners and their scholarship to improve your environment.
Article: Addressing racism through ownership. Dutt, K. DOI: 10.1038/s41561-021-00688-2
Article: Black Scientists Are Not the Door to Diversity. Hayes, CA. DOI: 10.1021/acschemneuro.1c00375
Article: The Burden of Service for Faculty of Color to Achieve Diversity and Inclusion: The Minority Tax. Trejo J. DOI: 10.1091/mbc.E20-08-0567
Summary: Marginalized people are expected to dismantle oppressive systems that actively disenfranchise them, an expectation known as the Minority Tax. How does this tax impact people at different stages of their career, and how do we combat this expectation?
Key Points:
- Successful DEIJ work takes teamwork.
- There is a lack of respect for DEIJ work.
- This is most obvious in the deliberate exclusion of DEI work from traditional metrics of professional progress, such as promotion and funding.
- Universities are often recognized and praised for the contributions of individuals to DEIJ work.
- There is a lack of support for DEIJ work.
- This work is rarely done by an expert. Instead, those with lived experiences are often tasked with developing and implementing DEI efforts. This model results in mixed outcomes while allowing universities to claim they support DEIJ initiatives.
Open Questions
- How do we get more people involved in DEIJ work?
- What do you do with people who are not interested in contributing to DEIJ?
- How do we track and reward DEIJ effort among academic personnel?
- How do we evaluate the impact of diversity efforts in academia?
- Is it appropriate for basic scientists to create and lead DEIJ efforts?
Proposed Action Items:
- Estimate how much time you are spending on DEIJ work compared to others. Take note of who shows up to meetings, comes up with ideas, and executes those ideas.
- Increase the importance of service work, specifically DEIJ work, in tenure and promotion decisions.
- Provide material resources, such as hiring full-time staff or providing money for consultants, to implement DEIJ projects or initiatives.
Background
A group of scientists within the Fraser lab have begun a journal club centered around issues of diversity, equity, inclusion, and justice within academia, specifically in the biological sciences.
Our goal is to provide an environment for continued learning, critical discussion, and brainstorming action items that individuals and labs can implement. Our discussions and proposed interventions reflect our own opinions based on our personal identities and lived experiences, and may differ from the identities and experiences of others. We will recap our discussions and proposed action items through a series of blog posts, and encourage readers to directly engage with DEIJ practitioners and their scholarship to improve your environment.
Article: The limits of settlers’ territorial acknowledgments. Asher L, Curnow J & Davis A (2018) DOI: 10.1080/03626784.2018.1468211
Summary: There has been an increase in the performance of land acknowledgments by non-Indigenous people in non-Indigenous, primarily academic settler, spaces. This article examines what purpose do these land acknowledgments serve, who are they for, and can land acknowledgments performed by settlers be improved to better reflect the original intentions of Indigenous people who created this practice?
Key Points:
- Settlers perform land acknowledgments as a means of combating the erasure of Indigenous people.
- The pedagogical intention has been to combat erasure and force settlers to grapple with our positionality.
- In becoming standardized and mainstream, it is reduced to a “mundane “box-ticking” exercise, easily ignored and void of learning opportunities.”
- Settler moves to innocence are those strategies or positionings that attempt to relieve the settler of feelings of guilt or responsibility without giving up land or power or privilege, without having to change much at all.
- The practice needs to be improved to avoid becoming rote and normalized.
Open Questions:
- What pedagogical work do territorial acknowledgments accomplish in settler spaces?
- What do people learn from a territorial acknowledgment and does it serve any decolonial purpose?
- Are territorial acknowledgments productive in disrupting avoidance mechanisms and pushing settlers towards decolonial solidarity?
Proposed Action Items:
- Begin including an intentional and well-researched land acknowledgment in your presentations.
- Include specific examples of how settlers can contribute to decolonial efforts.
Background
The PanDDA algorithm is a super useful tool for detecting low occupancy ligands in electron density maps obtained by X-ray diffraction. Low occupancy ligands are frequently encountered in fragment screening campaigns, and PanDDA can greatly increase the hit rate of a fragment screen and therefore increase the number of starting points available for fragment-based ligand discovery. We’ve used PanDDA for fragment screens against the PTP1B phosphatase and the NSP3 macrodomain from SARS-CoV-2. After modeling ligands, the data are deposited in the PDB. Data includes the atomic coordinates, the structure factor intensities, the map coefficients after final refinement with the ligand, and the PanDDA event map coefficients. The structure factor intensities and the map coefficients as separate data blocks in a single CIF.
The problems
There are two problems with looking at this data after downloading it from the PDB. The first problem is that because of the low occupancies of ligands, maps based on the structure factor intensities or the map coefficients after final refinement with the partial occupancy ligand often do not contain convincing electron density evidence for the bound ligand. That evidence is best found in the PanDDA map.
The second problem is that CIFs with multiple data blocks can be tricky to convert into MTZ files for visualization in COOT. From my experience, running phenix.cif_as_mtz will lead to the correct conversion of the map coefficients from the refined data, however, the PanDDA event map coefficients may not be converted. The structure factors encoding the PanDDA event map are based on the real space analysis and in space group P1, not the symmetry of the corresponding PDB file.
The solution
We split the CIF containing the three data blocks into separate CIFs. Actually, it’s fine just to extract the PanDDA event map block into one CIF, and move the original and refined data in another. Then run phenix.cif_as_mtz on the separate CIFs, with the correct symmetry flags specified, to convert them into MTZ files.
The extract_pandda.sh script does this for you. Download the coordinates and structure factor file from the Protein Data Bank (xxxx.pdb and xxxx-sf.cif files, where xxxx is the four letter PDB code) and move them to a working directory. On the command line, run ./extract_pandda.sh xxxx-sf.cif SG
(where SG is the space group of the crystal). The script will split the CIF into two separate CIFs, containing the refined and original data (xxxx-sf_data.cif) or the PanDDA event map (xxxx-sf_pandda.cif). The script then runs phenix.cif_as_mtz and converts the CIFs to MTZs for visualization in COOT.
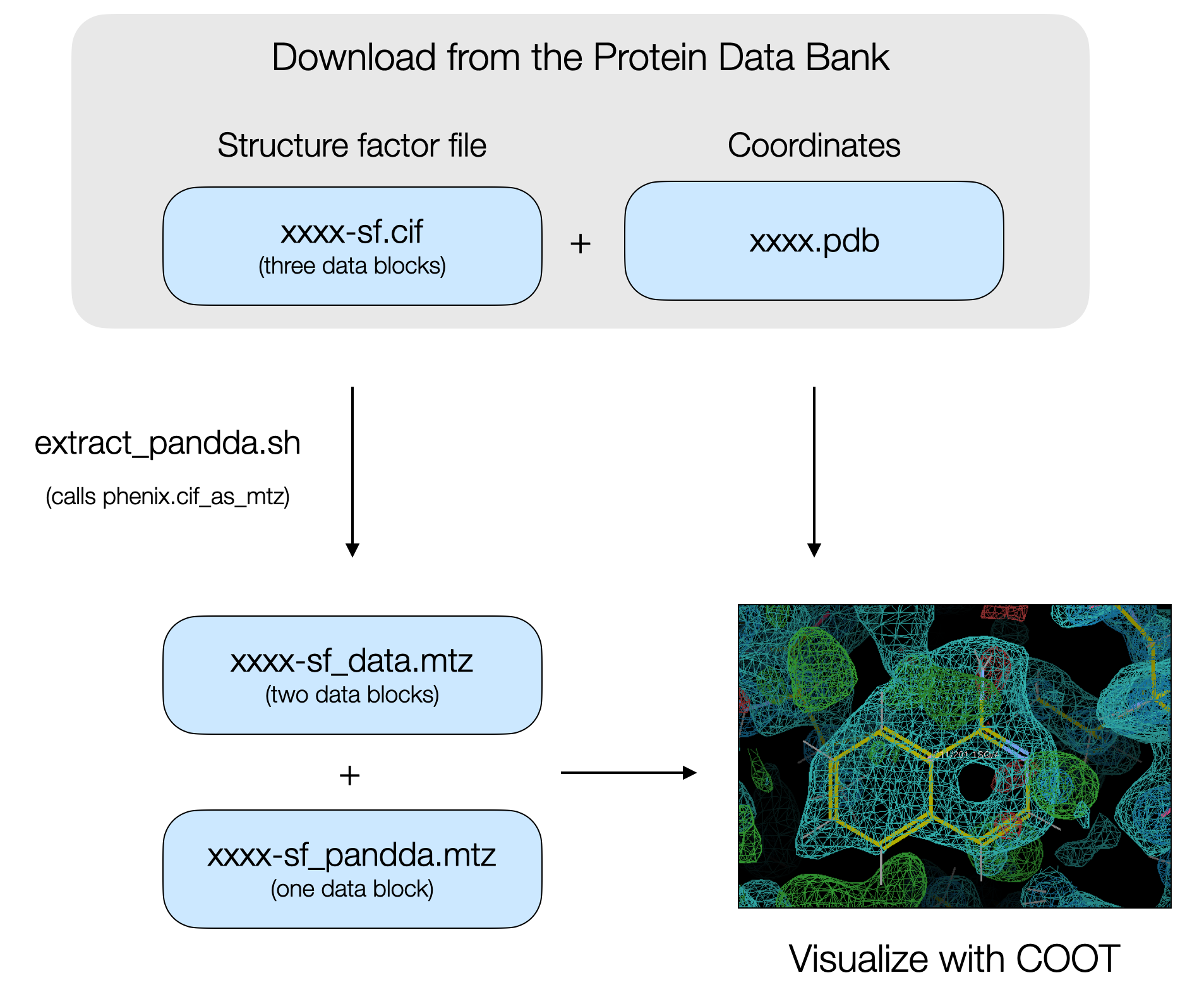
Caveats
The script needs the Phenix version dev-4338 to run, available here.
Data blocks need to be named as follows in the CIF (where xxxx is the PDB code):
- Data from final refinement with ligand: data_rxxxxsf
- PanDDA event map: data_rxxxxAsf
- Original data: data_rxxxxBsf